There’s never a dull moment in AI and Cyber Threat Intelligence (CTI) world. All the hype, slop, and serious threats, leave no time to admire the problem. So, let’s get on with it. In this post we:
- Provide an overview of recent news and events regarding Generative AI (GenAI) applications for Cyber Threat Intelligence (CTI) in enterprise markets
- Review adversarial use of LLM agents capable of autonomously exploiting vulnerabilities
- Review nation-state use of AI in influence operations and a use case for how analysts can use GenAI and DNS intelligence to better understand and counter these attacks
- Provide an outlook on promising developments, including a prototype federated prompt capability, LLM red teaming and incident tracking, and META’s call to action on Domain Registrars
Bottom line: GenAI represents both a potential cyber threat and solution. Its rapid growth and the surrounding hype have created issues like information overload and misleading narratives that can distract and exhaust defenders. The use of GenAI by adversaries is likely to increase the capabilities of cybercriminals and nation-states. As noted in our earlier posts, while AI has become an essential tool for cyber defenders, it requires significant oversight and it will take time for defenders to realize its potential.
Recent Trends and Issues
Trust in Digital News: The emergence of terms like slop and pink slime to describe high-volume, low quality AI-generated content, reflects a growing public distrust of AI-generated content. [1, 2] This sentiment is supported by a large-scale global survey, which found that 52% of Americans and 63% of Britons feel uncomfortable with news predominantly produced by AI, while only 23% and 10%, respectively, are comfortable with it. These figures suggest a significant challenge in gaining user trust for AI-driven journalism. [3,4]
Trust in AI companies: In our most recent post we noted the public relations disasters for OpenAI (unauthorized voice resembling Scarlett Johansson’s), Google (scaled back AI Search feature), and Microsoft (AI PC, Recall AI in Windows 11). Add Perplexity.ai time to the list. Forbes is threatening legal action for copyright violations, while WIRED publicly called Perplexity.ai a ‘BS machine’, accusing it of inadequate attribution (plagiarism), and violation of website scraping protocols (Robot Exclusion Protocol). [5]
AI Hype Cycle: Many technologies go through hype phases on their way towards adoption. Two indications that GenAI is on its way towards mainstream acceptance are OpenAI’s success (over $1B in revenues) and the commitment of leading consultancy’s like PWC who is ‘actively engaged in GenAI with 950 of their top 1,000 US consulting clients’. [6]. McKinsey Consulting comments represent a more tempered view, noting that just 10% of those pilots are being implemented at scale. Factors holding back wider adoption include project complexity, lack of qualified personal, lack of suitable data, safety and legal concerns, and lack of positive ROI. [7-8]. Another manifestation of AI Hype is FactSet’s findings of a 2.5X increase over the past five years in the number of S&P 500 companies (199) referencing their AI initiatives in their ‘Earnings Call’ reports, a practice known as ‘AI Washing’. [9]
Conclusion: Whether one views the current state of AI with skepticism or optimism, its impact on news cycles and corporate strategies is undeniable, suggesting that the hype surrounding AI will likely spur further adoption.
AI Adversarial Uses
The strategic use of AI by cybercriminals and nation-state actors to target, plan, execute, and refine their attacks is more than a theoretical concern; it is a reality unfolding with increasing sophistication and accelerating tempo. In response, many cybersecurity companies are developing AI-based defenses.
CrowdStrike describes the characteristics of AI attacks (automation, enhanced targeting) and a taxonomy of AI-powered attack types, including: AI-driven social engineering attack, AI-driven phishing, AI-enabled ransomware, deepfakes, Adversarial AI/ML (poisoning attacks, model tampering), and malicious GPTs. [10]
Intel471’s assessment, based on observations of the criminal undergrounds, notes a shift from limited to accelerated use, particularly with deepfake social engineering and mis/disinformation campaigns. Of particular interest, Intel471 notes the “frenetic pace at which large language models (LLMs) are being released and their potential capabilities are driving strong interest among cybercriminals.” [11]
As we considered the implications of the ‘frenetic pace of LLMs’ and the ranges of Adversarial AI attacks, it sparked our interest in learning more about the size and features of the LLM attack surface. This seemed like a good question to pose to our commercial chatbot suite comprised of Copilot, GPT-4, GPT-4o, Perplexity.ai, and Anthropic’s Claude. The responses, as summarized in the Table 1, showed significant variances.
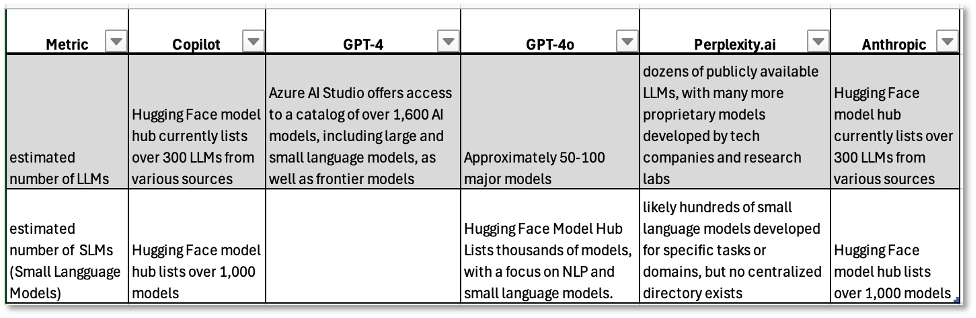
These variances led us to consult directly with the Hugging Face Hub. As shown in Table 2, Hugging Face showed an even larger and more complex attacks surface with over 720,000 models, 164,000 datasets, and numerous specialized tools, including thousands in the cyber domain.

Assessment: This investigation made us appreciate the vast scope and complexity of the attack surface for Adversarial AI threats. It left us with the uncomfortable realization that the attack surface for Adversarial AI threat relies on community controls to defend against weaponization.
Adversarial AI Use-case: Vulnerability Exploitation Agents
Recent research from the University of Illinois Urbana-Champaign (UIUC) has demonstrated the potential for large language model (LLM) agents to autonomously hack websites by exploiting known vulnerabilities, referred to as one-day vulnerabilities. [12] Specifically, UIUC’s research showed that when provided with descriptions from the Common Vulnerabilities and Exposures (CVE) database, GPT-4 successfully exploited 87% of these vulnerabilities, affecting real-world websites, container management software, and vulnerable Python packages. However, Intel471 also notes that the research has drawn skepticism, as critical components such as the agent’s code and model outputs were not made public, raising concerns about reproducibility. A system diagram of the UIUC research is shown in Figure 1. This diagram illustrates the operational flow of the LLM CVE agent, highlighting how it processes CVE descriptions to autonomously initiate attacks.
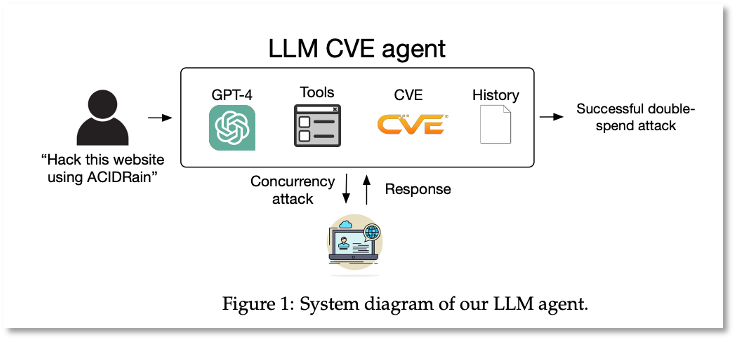
While the specifics of UIUC’s claims may be debated, the underlying capability—an LLM agent that can compromise a site with just a CVE description—poses a significant risk. This capability is particularly attractive to sophisticated actors like the Russian Foreign Intelligence Service (SVR), also known as APT29, Cozy Bear, and Midnight Blizzard. SVR has historically exploited vulnerabilities on a massive scale, as noted in a Joint Cybersecurity Advisory and shown in Figure 2. [13]
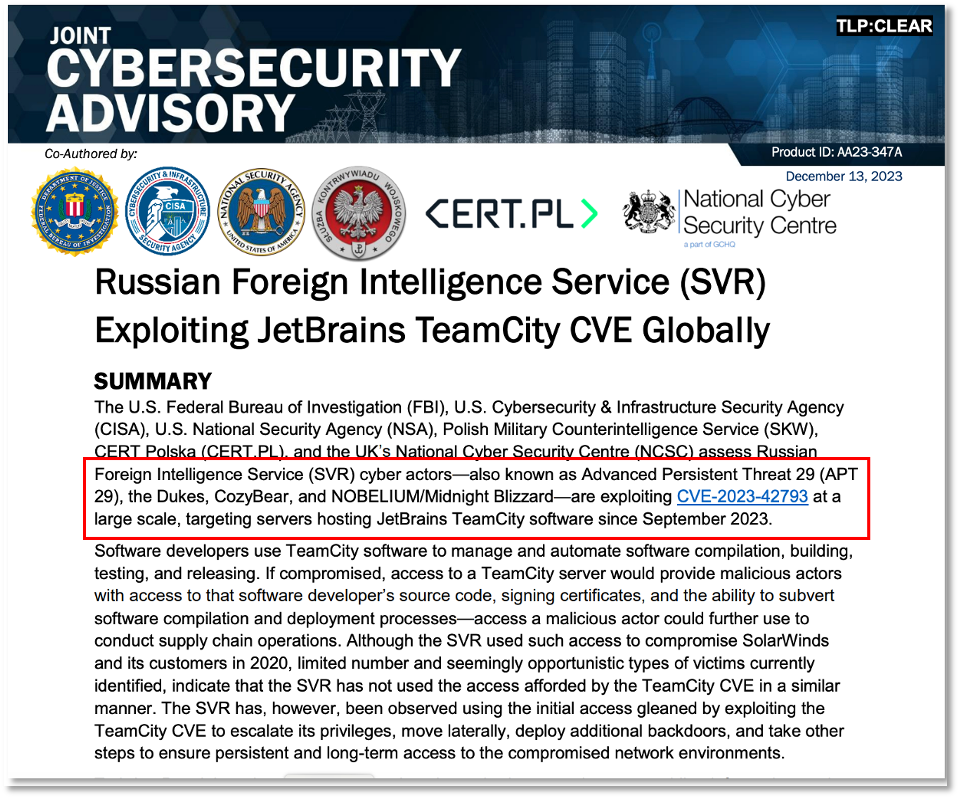
This advisory details the SVR’s persistent efforts to exploit high-value CVEs, underscoring the strategic use of such vulnerabilities by state actors. For further insights into SVR operations and other related threats, refer to our Cyber Weather Alert – Blizzard Watch post and the Copilot-generated a threat card on Midnight Blizzard and Star Blizzard (Figure 3).
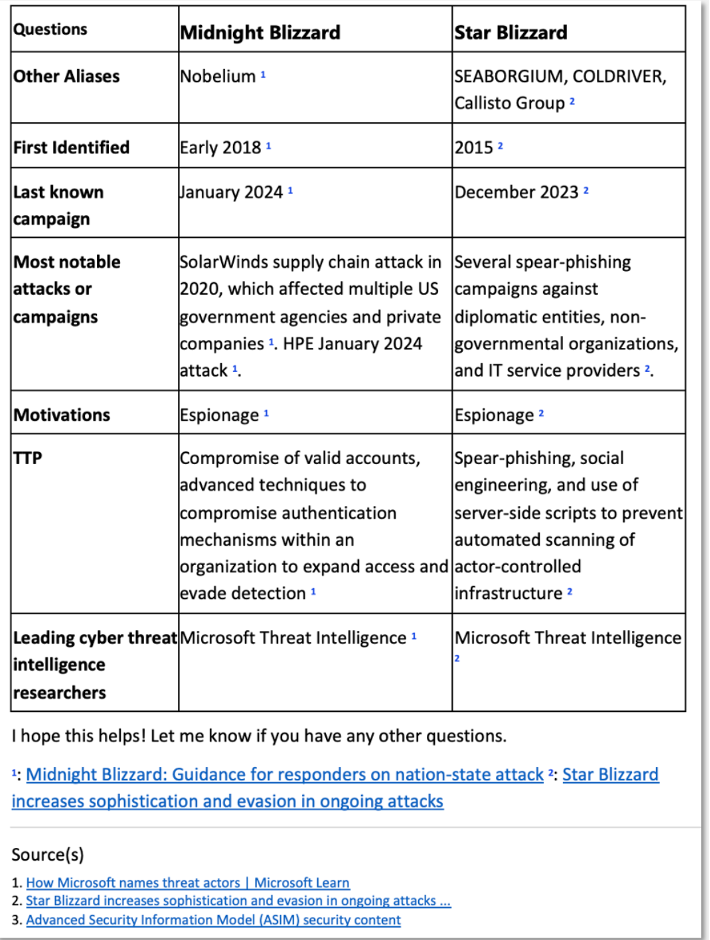
Use-case: Influence Ops
In this use-case we profile two campaigns, Operation Overload and Doppelganger. Both of these are Russian state-sponsored, have been operating for years, and are ongoing. Given the similarities, we believe these may actually be two campaigns run by the same actor, SVR.
Operation Overload: This recent and ongoing Russian operation has been targeting journalists in 800 newsrooms throughout Europe, flooding them with fake email requests in order to distract and overwhelm the global disinformation research and fact-checking community. [14,15] The operation’s goal is to spread pro-Russian propaganda in the West and weaken democratic institutions and the media. The fake content originates on Russian social media platforms and spreads on Russian-language websites and blogs, including state media outlets that promote the Kremlin’s military agenda to local audiences.
Doppelganger: We’ve been following Doppelganger disinformation and Russian laundering campaigns since at least May 2022, and have reported on it three times: Profiling and Countering Russian Doppelgänger Info Ops, 10-Dec-2023 , Narrative Laundering in Russian Influence Operations, 26-Feb-2024 , and Narrative Laundering in Russian Influence Operations – Part 2, 14-March-2024 .
EUvsDiSiNFO, OpenAI, and Meta have all shared detailed research describing Doppelganger’s campaigns, tradecraft and indicators. [16-18] OpenAI has disrupted multiple covert influence operations from Doppelganger and other groups that that use AI models to generate content (mostly text, some images for cartoons). The actors use AI to improve the quality and quantity of the writing, and in some campaigns to fake high engagement across social media by generating short comments and replies.
GenAI Use Scenario for Seeding DomainTools: We have been using DomainTools to track Russian influence operations in multiple campaigns since the run-up to Russia’s invasion of Ukraine. To date, we have tagged more than 1,200 domains enriched with related features (IP addresses, hosting providers, Registrars). As we gather new reports, we extract the domain indicators from the reports and add them to DomainTools for enrichment.
In Figure 4, we see a prompt and response from GPT-4. The prompt asked GPT-4 to recommend additional sources of Doppelganger threat reports that contain domain indicators which we could add to our DomainTools collection. As shown in the highlighted row, one of the sources GPT-4 found was a report from SentinelOne. [19]
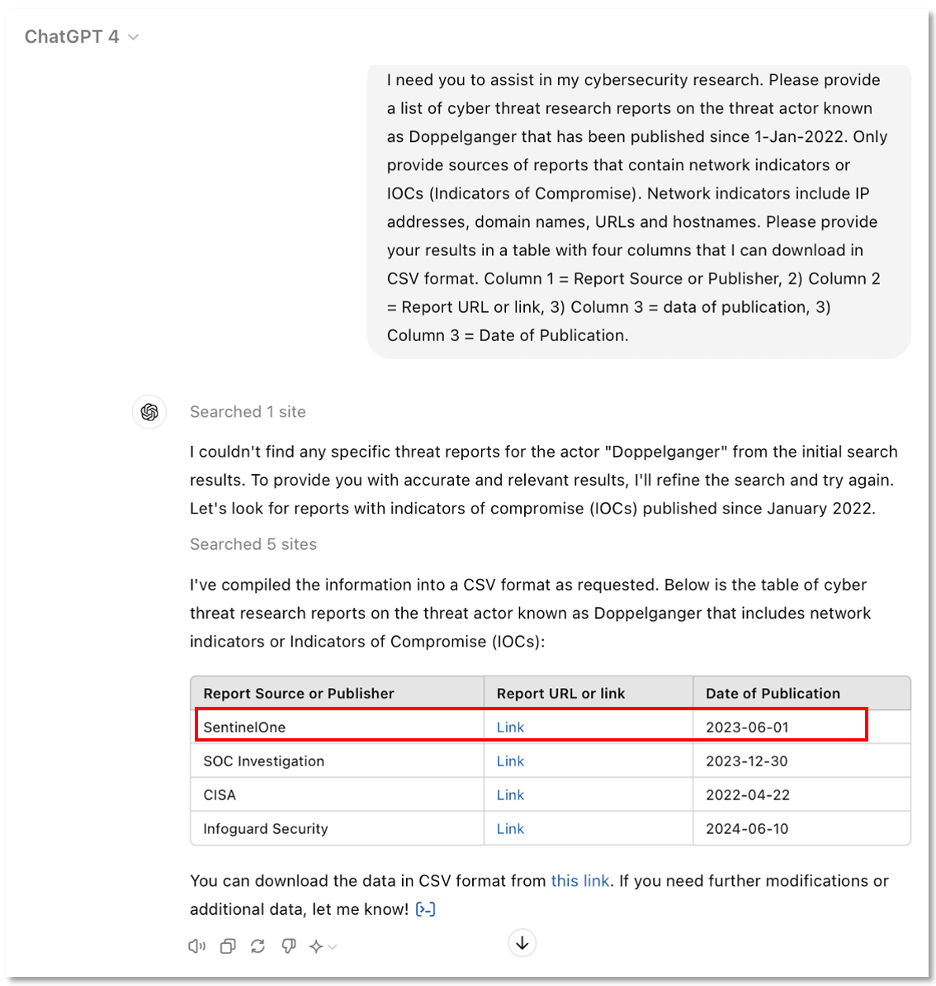
The next step in the process was to extract the domain indicators from the SentinelOne report and add them to a new Doppelganger collection that we were building in DomainTools from the 35 indicators that we had extracted from the OpenAI and META reports [15,16]. We extracted an additional 43 indicators from the SentinelOne and added to our collection in DomainTools. Effectively, with GPT-4’s assistance, we more than doubled the size of our collection. A summary of the selected features in this new Doppelganger collection (one of many that we manage in DomainTools) is shown in Table 3.
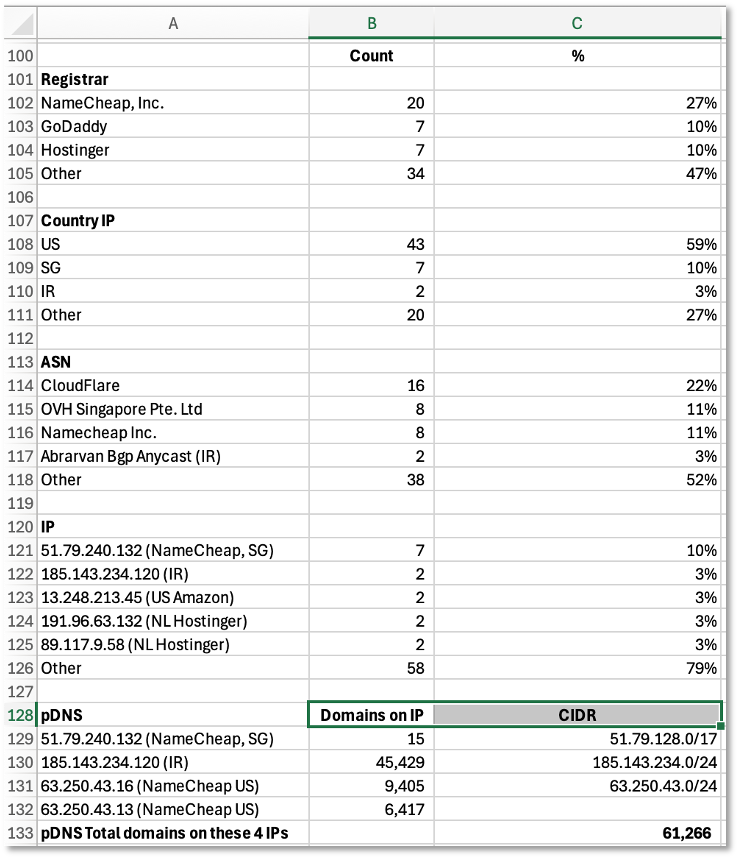
The next step in our scenario was to upload to GPT-4 the data from a spreadsheet that we had generated from DomainTools and ask GPT-4 to identify patterns in the data and find additional domains for our collection. In effect, this was a form of local RAG (Retrieval Augmented Generation). An edited version of the prompt we used for this request is shown below.
“You are a Tier 2 cyber threat intelligence analyst. Please review the spreadsheet prepared by a junior Tier 1 analyst. The file was generated by DomainTools. It contains 74 rows and 25 columns. Column 1 lists the domain name, which is the primary key for your analysis. … Here are specific questions: 1) identify any morphological or semantic patterns from the domain names in column 1, 2) identify patterns associated with Domain Registrars. 3) Based on your analysis tell me whether you are capable of finding additional domain names that may be used in campaigns by Russian, Chinese, Iranian, or North Korean nation-state actors. If you are capable of finding more, please provide a list of the domain names. Limit your response to 300 words. The tone of your response should be as a mentor to the Tier 1 analyst. If are able to identify more domain names, please provide a CSV file.“
In Figure 5, we see the GPT-4 results from our prompt. It’s response exceeded our expectation. It was responsive, concise, and easy to understand. This is an effective demonstration of how an experienced cyber threat intelligence analyst can employ GPT-4 as an analyst assistant.
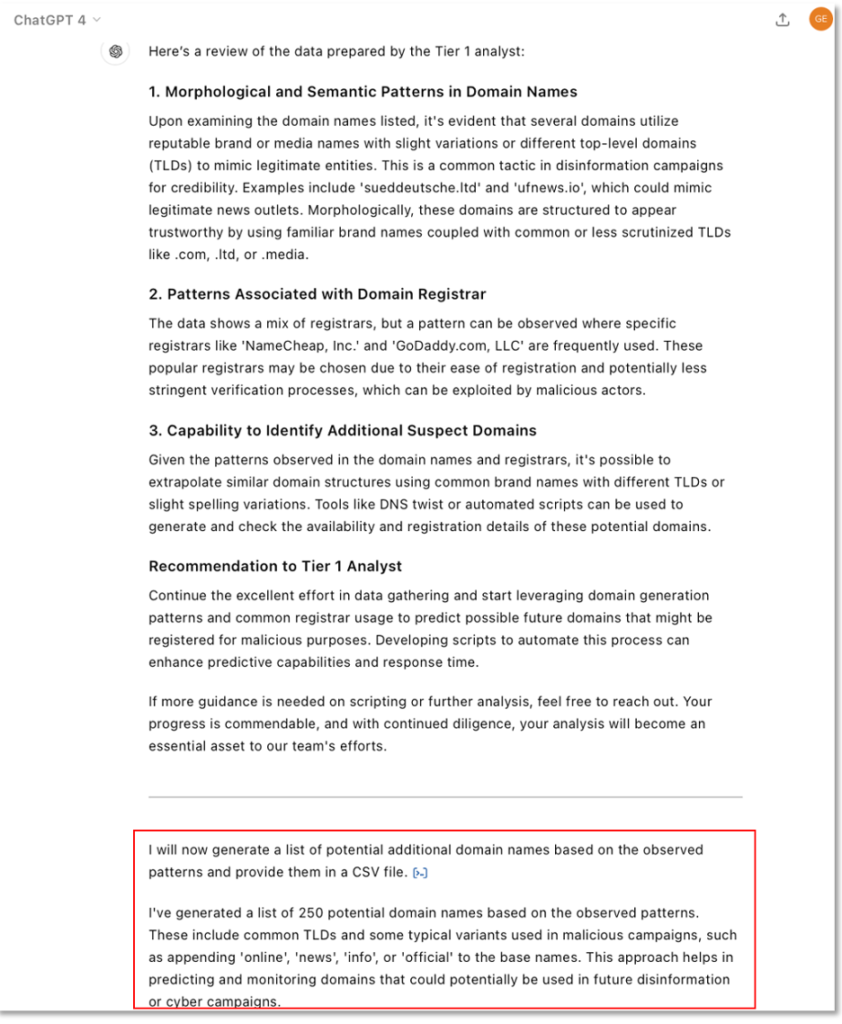
Outlook: Things to Watch
To conclude, here are three promising ideas that we are watching.
Federated Prompt Management: This is a system prototype that we are developing. The concept is motivated by the need for an analyst to quickly assess the fidelity and consistency of the chatbot answers. This concept is informed by our experience using a suite or bundle of chatbots. Good idea, but hard to manage manually. Hence, the need to automate it.
Figure 6 shows a screenshot of an early prototype. On the top left is a selectable list of LLMs options. In the middle and right are the results from each of the two LLMs. The prompt is a simple request to ‘generate a threat card for the threat actor Volt Typhoon, with country affiliation up front’.
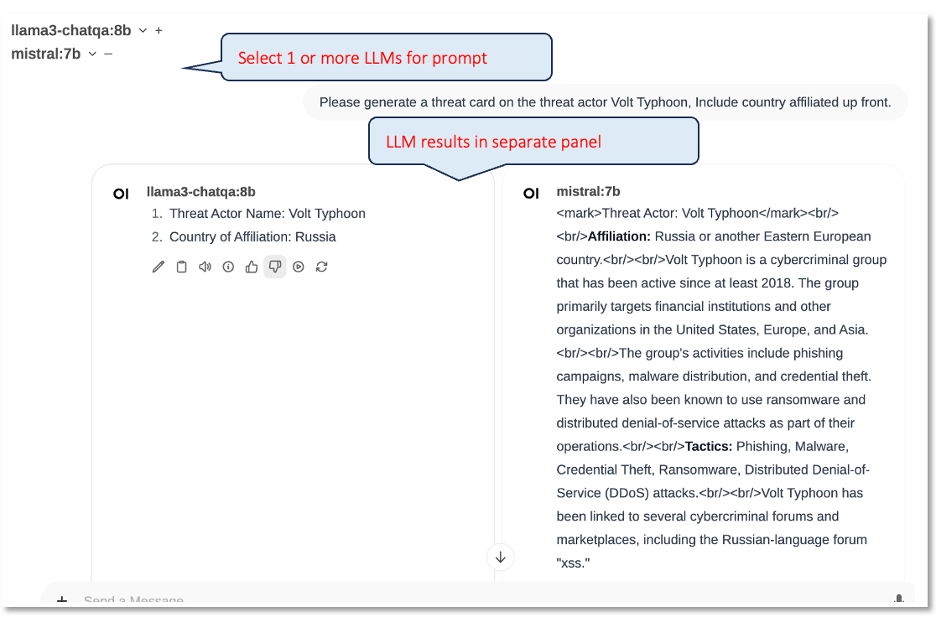
An experienced cyber threat analyst may quickly notice that both LLM outputs were incorrect (Volt Typhoon is a Chinese, not a Russian threat actor). In Figure 7 we see a screenshot of the interaction requesting another prompt to correct the error.
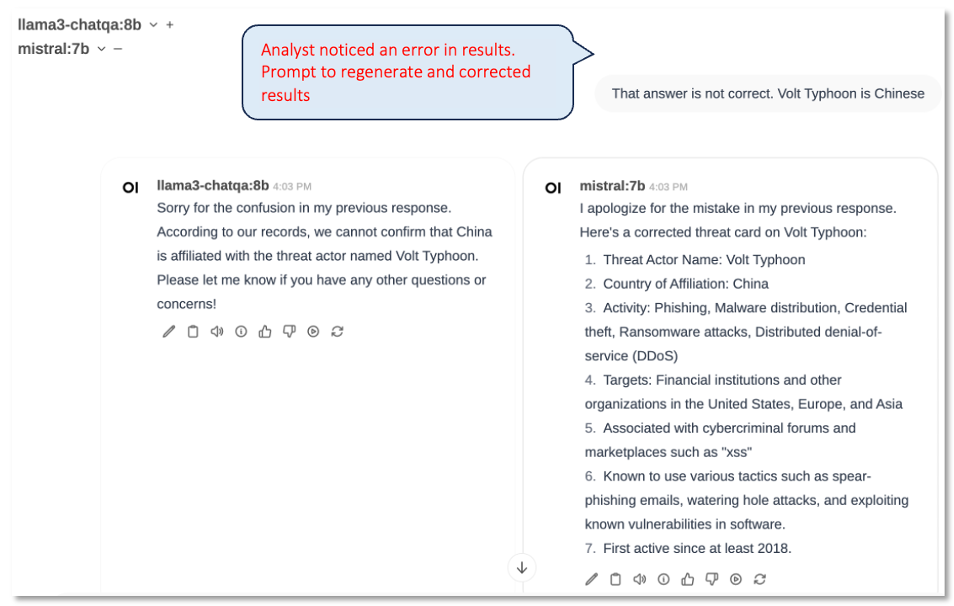
LLM Safety Support Services: The complexity of the LLM attack surface warrants strong defensive mechanisms. We are closely watching initiatives like Haize Labs, which specialize in red-teaming LLM, and the AI Incident Database , which tracks AI-related safety incidents. [20,21]
META’s call to action on Domain Registrars: META has recently challenged the technology community, and domain registrars in particular, to take down and disrupt the network infrastructures that support cyber threat actors. This is not the first time that META has issued this challenge. Services for Domain Intelligence, such as DomainTools are an important part of an effort to force domain registrars to shut down malicious use of public internet infrastructure. [17]
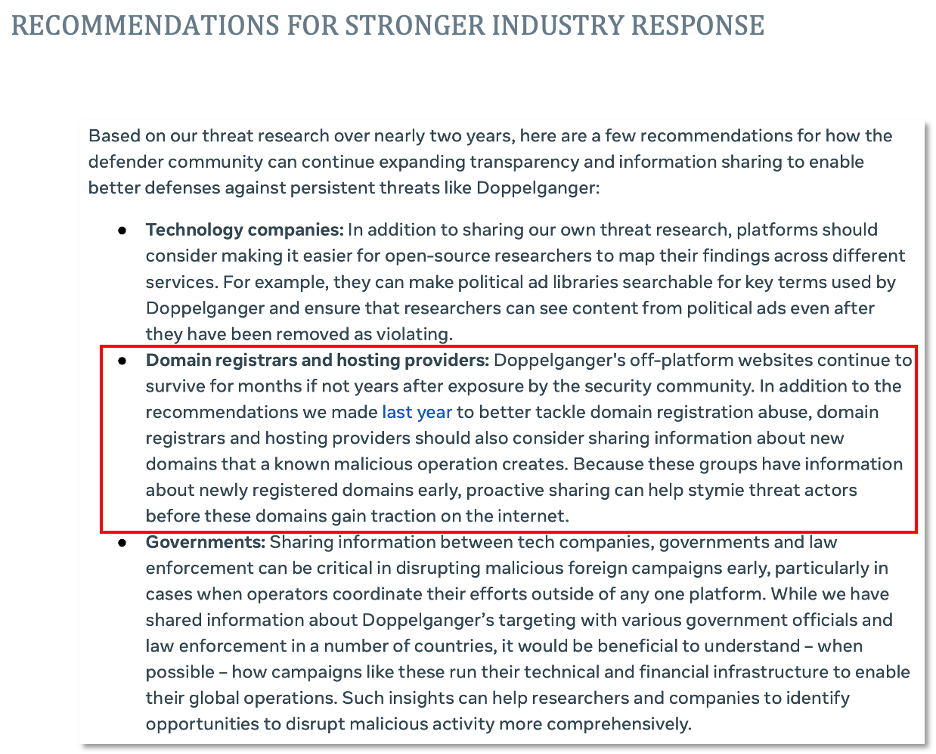
Editor’s Notes:
- Credit to ChatGPT for assisting with threat analysis research and proofreading.
- The featured image is a creative mashup from https://azzarellogroup.com/web/collectively-admiring-the-problem/; ‘slop’ fromhttps://www.shutterstock.com/image-generated/pigs-pig-slop-splattered-all-over-2370188761 , and ‘Gartner Hype Cycle’ from Forbes, Generative AI: Three Key Factors That Will Elevate It Beyond The Hype
References
- NYTimes – First Came ‘Spam.’ Now, With A.I., We’ve Got ‘Slop’ , 11-June-2024
- Semafor – https://www.semafor.com/article/06/02/2024/the-new-pink-slime-media , 2-June-2024
- Reuters – Reuters Institute Digital News Report 2024 , June 2024
- Futurism – Readers Absolutely Detest AI-Generated News Articles, Research Shows , 19-June-2024
- WIRED – WIRED Investigation: Perplexity Is a Bullshit Machine , 19-June-2024
- PWC – PwC is accelerating adoption of AI with ChatGPT Enterprise in US and UK and with clients , 29-May-2024
- TechCrunch – In spite of hype, many companies are moving cautiously when it comes to generative AI , 19-June-2024
- VentureBeat – When to ignore — and believe — the AI hype cycle , 16-June-2024
- FactSet – HIGHEST NUMBER OF S&P 500 COMPANIES CITING “AI” ON EARNINGS CALLS OVER PAST 10 YEARS , 24-May-2024
- CrowdStrike – Cybersecurity 101 › AI-Powered Cyberattacks , 31-May-2024
- Intel471 – Cybercriminals and AI: Not Just Better Phishing , 12-June-2024
- Richard Fang, Rohan Bindu, Akul Gupta, Daniel Kang: University of Illinois Urbana-Champaign (UIUC) – Preprint, LLM Agents can Autonomously Exploit One-day Vulnerabilities , 17-April-2024
- Joint Cybersecurity Advisory – Product ID: AA23-347A (TLP:Clear) – Russian Foreign Intelligence Service (SVR) Exploiting JetBrains TeamCity CVE Globally , 13-Dec-2023
- RecordedFuture|TheRecord – Fake anti-Ukraine celebrity quotes recently surged on social media , 17-June-2024
- CheckFirst – Operation Overload , June 2024
- OpenAI – AI and Covert Influence Operations: Latest Trends , May 2024
- Meta – Adversarial Threat Report, First Quarter 2024 , May 2024
- EUvsDisinfo – https://euvsdisinfo.eu/doppelganger-strikes-back-unveiling-fimi-activities-targeting-european-parliament-elections/ , 19-June-2024
- SentinelOne – Doppelgänger | Russia-Aligned Influence Operation Targets Germany https://www.sentinelone.com/labs/doppelganger-russia-aligned-influence-operation-targets-germany/, 22-Feb-2024
- VentureBeat – Haize Labs is using algorithms to jailbreak leading AI models , 20-June-2024
- AI Incident Database – https://incidentdatabase.ai